Recent studies show that advanced priors play a major role in deep generativemodels. Exemplar VAE, as a variant of VAE with an exemplar-based prior, hasachieved impressive results. However, due to the nature of model design, anexemplar-based model usually requires vast amounts of data to participate in training, which leads to huge computational complexity. To address this issue, wepropose Bayesian Pseudocoresets Exemplar VAE (ByPE-VAE), a new variant of VAE with a prior based on Bayesian pseudocoreset. The proposed prior is condi.tioned on a small-scale pseudocoreset rather than the whole dataset for reducingthe computational cost and avoiding overfitting. Simultaneously, we obtain theoptimal pseudocoreset via a stochastic optimization algorithm during VAE trainingaiming to minimize the Kullback-Leibler divergence between the prior based onthe pseudocoreset and that based on the whole dataset. Experimental results showthat ByPE-VAE can achieve competitive improvements over the state-of-the-artVAEs in the tasks of density estimation, representation learning, and generativedata augmentation. Particularly, on a basic VAE architecture, ByPE-VAE is up to 3times faster than Exemplar VAE while almost holding the performance. Code isavailable at https://github.com/Aiqz/ByPE-VAE.
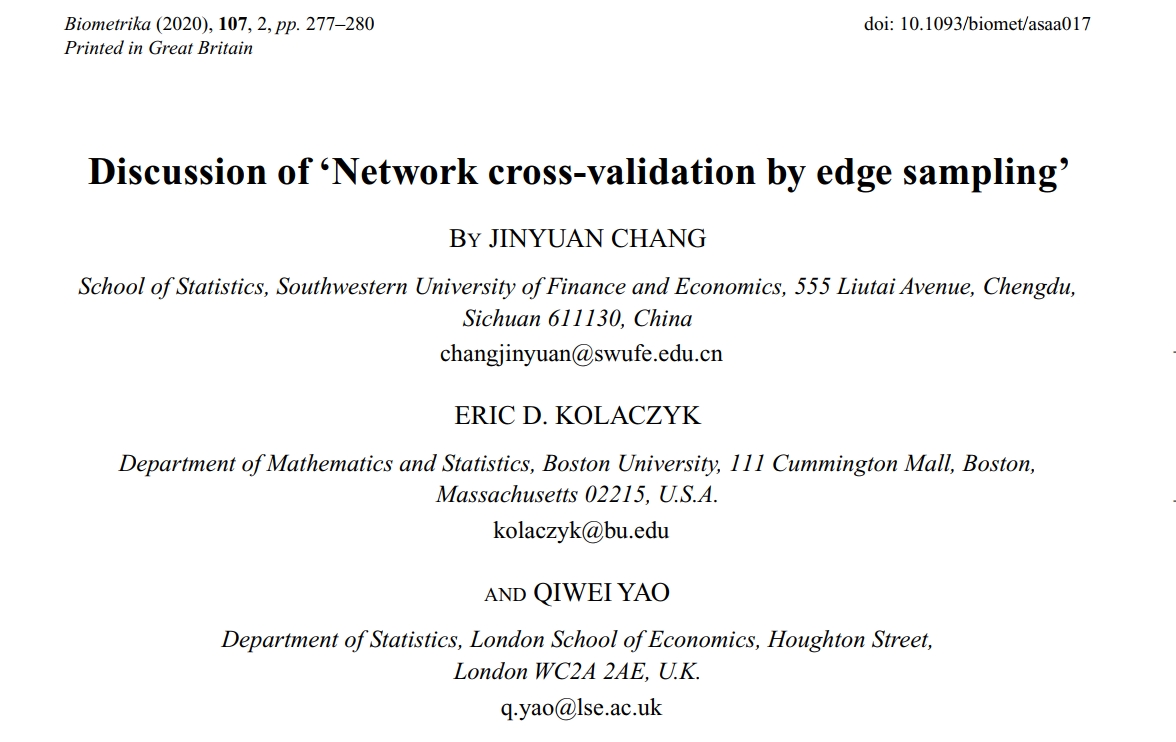