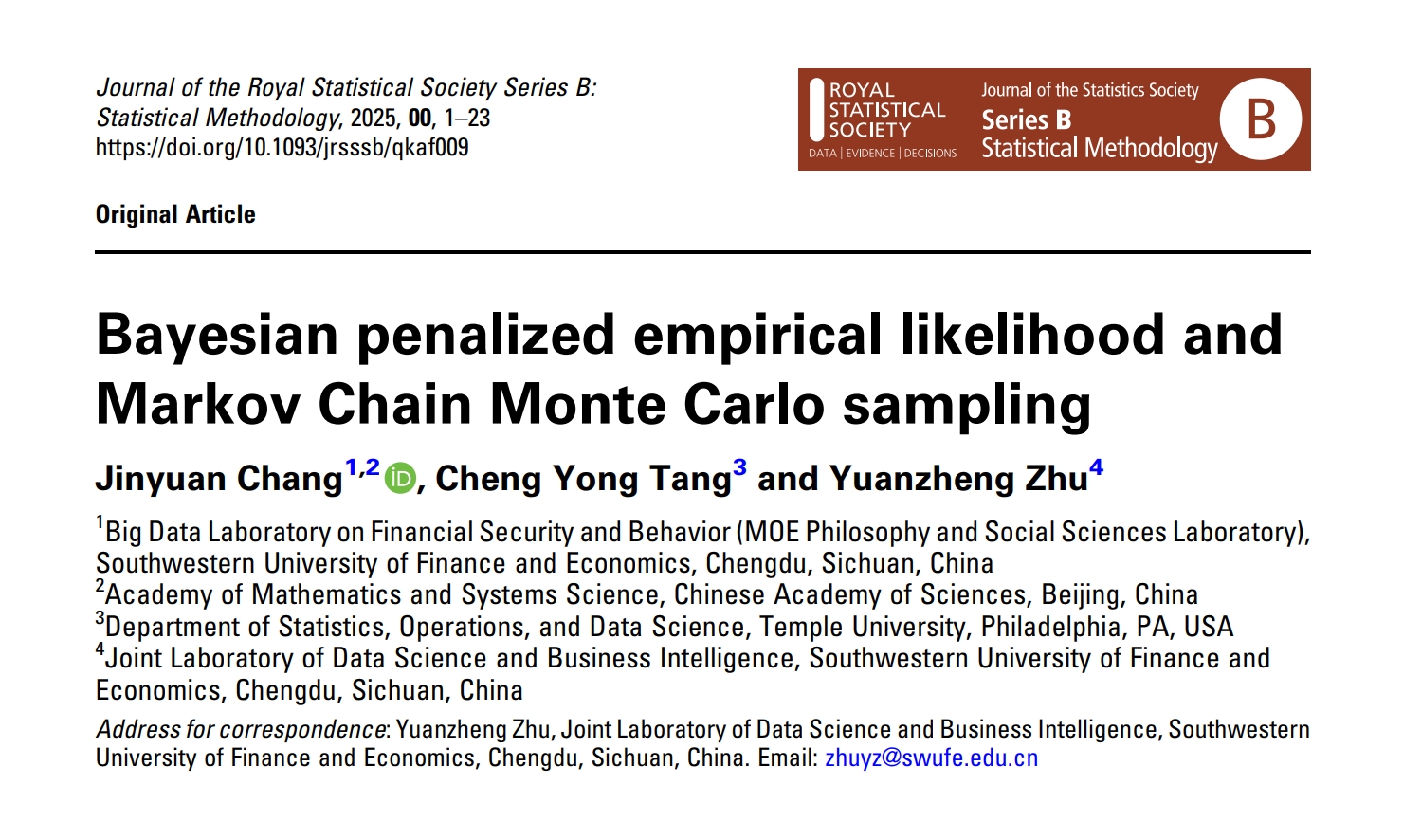
Chang, J., Tang, C. Y., & Zhu, Y. (2025+). Bayesian penalized empirical likelihood and Markov Chain Monte Carlo sampling. Journal of the Royal Statistical Society Series B, in press.
We propose a two-step procedure to model and predict high-dimensional functional time series, where the number of function-valued time series p is large in relation to the length of time series n. Our first step performs an eigenanalysis of a positive definite matrix, which leads to a one-to-one linear transformation for the original high-dimensional functional time series, and the transformed curve series can be segmented into several groups such that any two subseries from any two different groups are uncorrelated both contemporaneously and serially. Consequently in our second step those groups are handled separately without the information loss on the overall linear dynamic structure. The second step is devoted to establishing a finite-dimensional dynamical structure for all the transformed functional time series within each group. Furthermore the finite-dimensional structure is represented by that of a vector time series. Modeling and forecasting for the original high-dimensional functional time series are realized via those for the vector time series in all the groups. We investigate the theoretical properties of our proposed methods, and illustrate the finite-sample performance through both extensive simulation and two real datasets. Supplementary materials for this article are available online, including a standardized description of the materials available for reproducing the work.