Graph convolutional networks (GCN) are viewed asone of the most popular representations among thevariants of graph neural networks over graph dataand have shown powerful performance in empiricalexperiments. That l2-based graph smoothing enforces the global smoothness of GCN, while (soft)l1-based sparse graph learning tends to promotesignal sparsity to trade for discontinuity. This paper aims to quantify the trade-off of GCN betweensmoothness and sparsity, with the help of a generall, ℓp-regularized (1 < p≤ 2) stochastic learning pro.posed within. While stability-based generalizationanalyses have been given in prior work for a secondderivative objectiveness function, our ℓp-regularized learning scheme does not satisfy such a smooth con.dition. To tackle this issue, we propose a novel SGD proximal algorithm for GCNs with an inexactoperator. For a single-layer GCN, we establish anexplicit theoretical understanding of GCN with the ℓp-regularized stochastic learning by analyzing thestability of our SGD proximal algorithm. We conduct multiple empirical experiments to validate ourtheoretical findings.
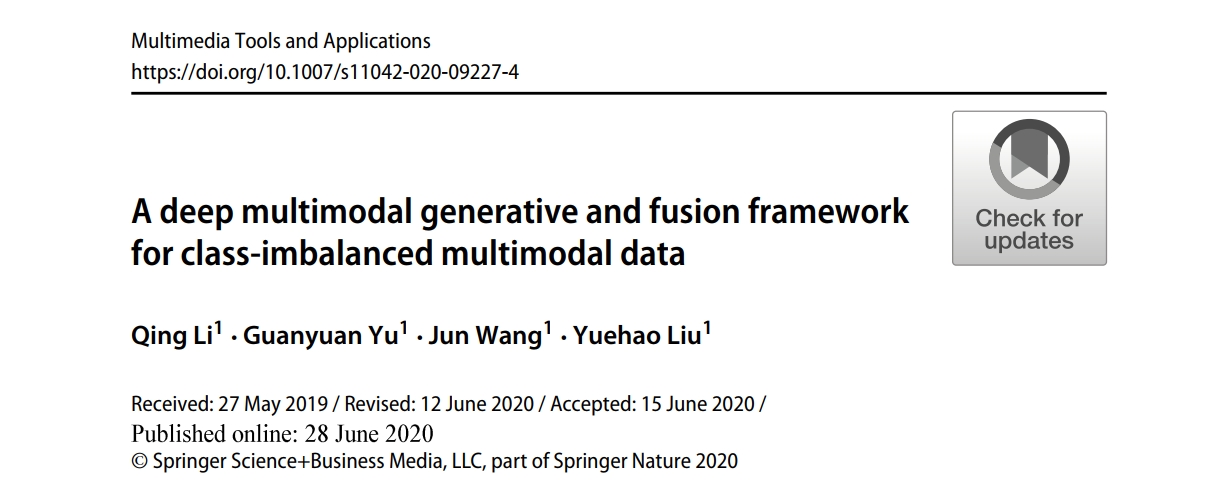