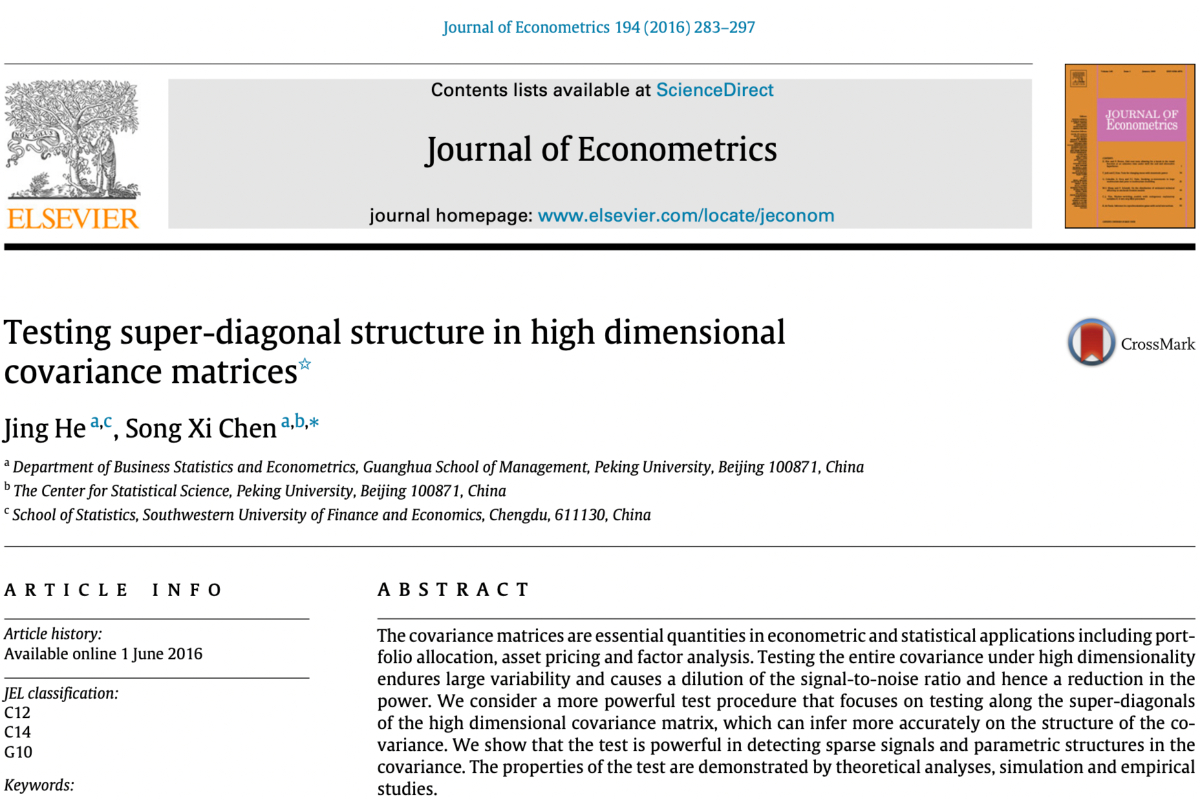
何婧, & Chen, S. X. (2016). Testing super-diagonal structure in high dimensional covariance matrices. Journal of Econometrics, 194, 283-297.
The covariance matrices are essential quantities in econometric and statistical applications including port- folio allocation, asset pricing and factor analysis. Testing the entire covariance under high dimensionality endures large variability and causes a dilution of the signal-to-noise ratio and hence a reduction in the power. We consider a more powerful test procedure that focuses on testing along the super-diagonals of the high dimensional covariance matrix, which can infer more accurately on the structure of the co- variance. We show that the test is powerful in detecting sparse signals and parametric structures in the covariance. The properties of the test are demonstrated by theoretical analyses, simulation and empirical studies.