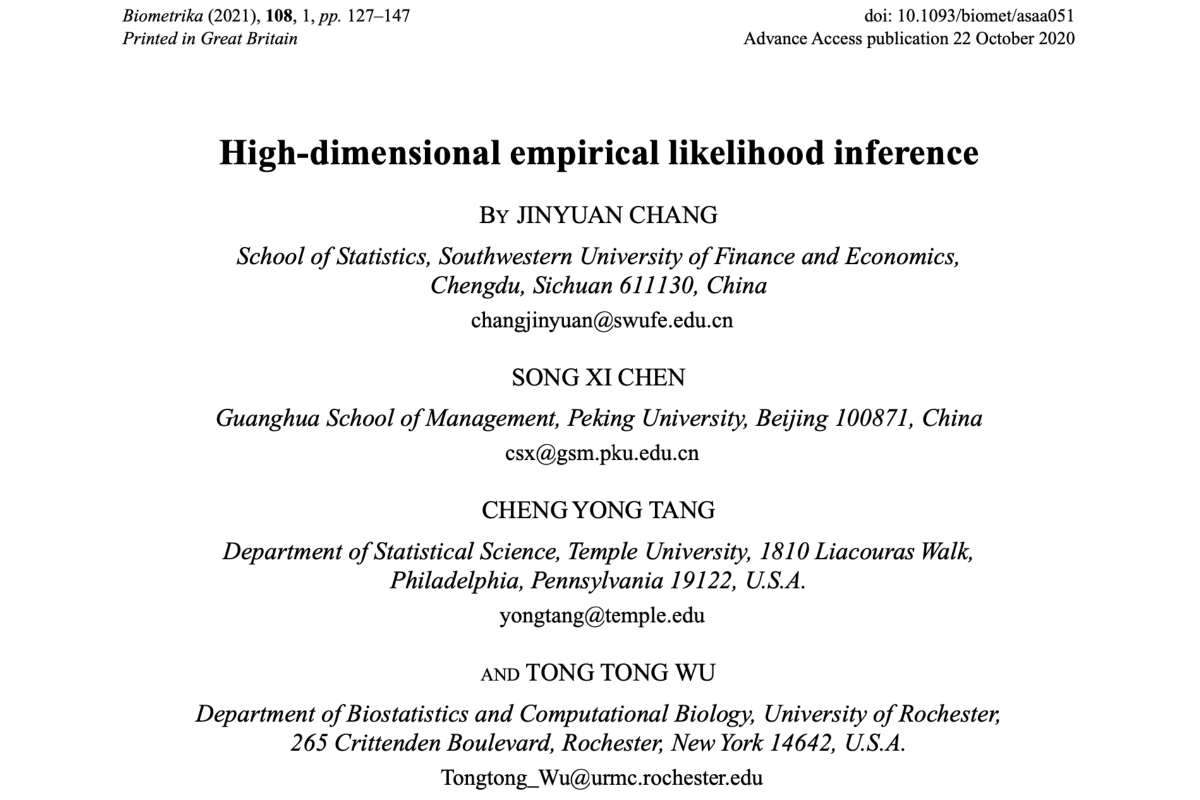
常晋源, Chen, S. X., Tang, C. Y., & Wu, T. T. (2021). High-dimensional empirical likelihood inference. Biometrika, 108, 127-147.
High-dimensional statistical inference with general estimating equations is challenging and remains little explored. We study two problems in the area: confidence set estimation for multiple components of the model parameters, and model specifications tests. First, we propose to construct a new set of estimating equations such that the impact from estimating the high-dimensional nuisance parameters becomes asymptotically negligible. The new construction enables us to estimate a valid confidence region by empirical likelihood ratio. Second, we propose a test statistic as the maximum of the marginal empirical likelihood ratios to quantify data evidence against the model specification. Our theory establishes the validity of the proposed empirical likelihood approaches, accommodating over-identification and exponentially growing data dimensionality. Numerical studies demonstrate promising performance and potential practical benefits of the new methods.