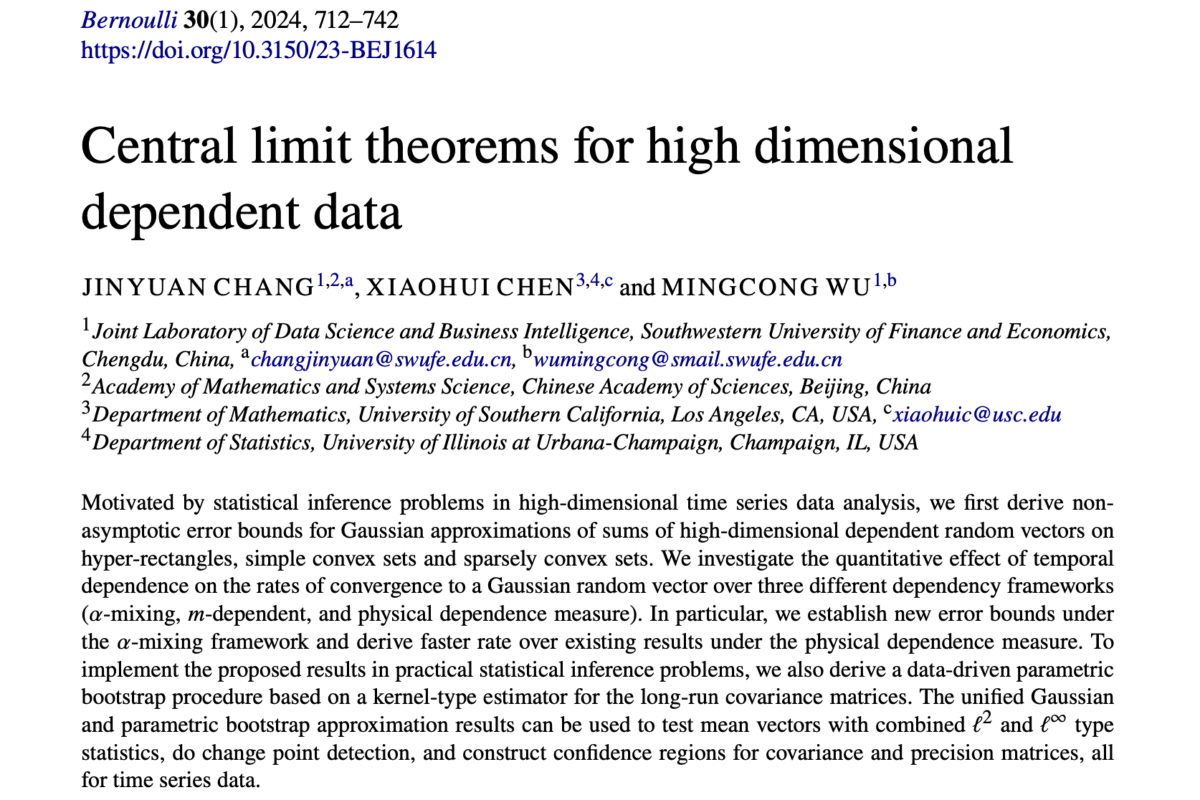
Chang, J., Chen, X., & Wu, M. (2024). Central limit theorems for high dimensional dependent data. Bernoulli, 30, 712-742.
Motivated by statistical inference problems in high-dimensional time series data analysis, we first derive non- asymptotic error bounds for Gaussian approximations of sums of high-dimensional dependent random vectors on hyper-rectangles, simple convex sets and sparsely convex sets. We investigate the quantitative effect of temporal dependence on the rates of convergence to a Gaussian random vector over three different dependency frameworks (α-mixing, m-dependent, and physical dependence measure). In particular, we establish new error bounds under the α-mixing framework and derive faster rate over existing results under the physical dependence measure. To implement the proposed results in practical statistical inference problems, we also derive a data-driven parametric bootstrap procedure based on a kernel-type estimator for the long-run covariance matrices. The unified Gaussian and parametric bootstrap approximation results can be used to test mean vectors with combined l2 and l∞ type statistics, do change point detection, and construct confidence regions for covariance and precision matrices, all for time series data.