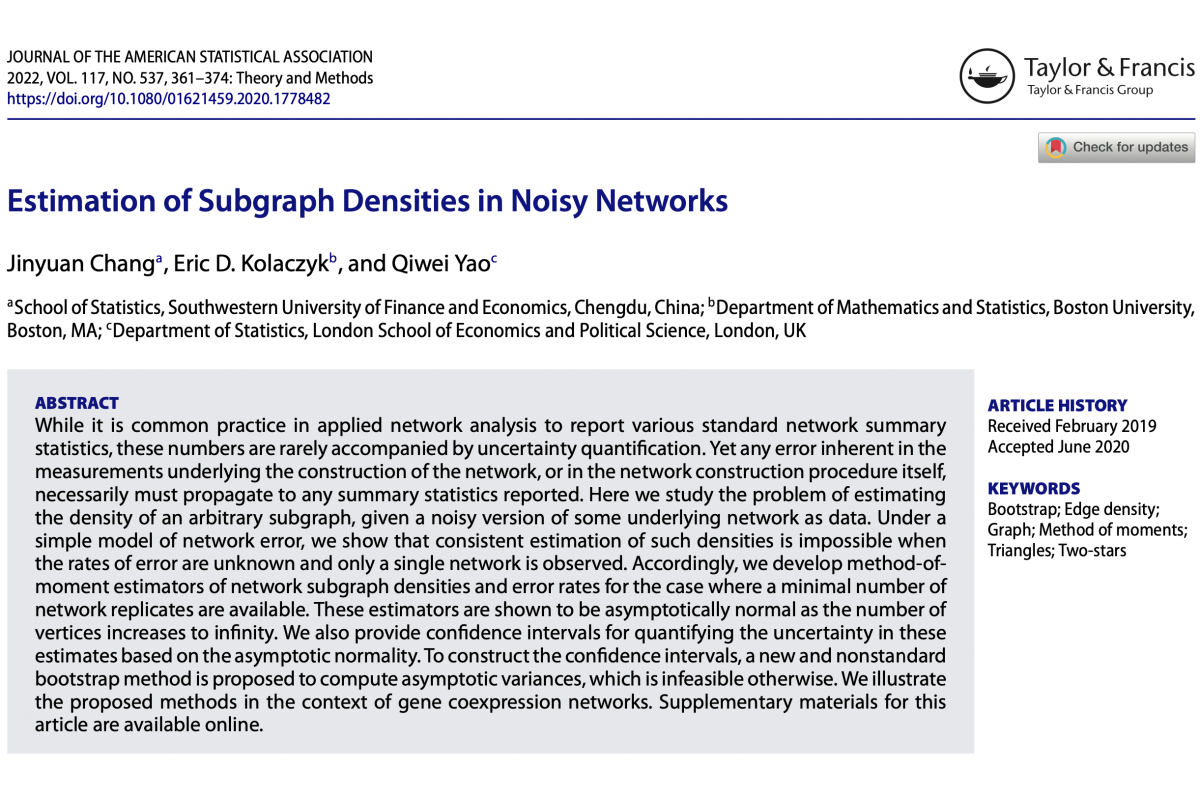
Chang, J., Kolaczyk, E. D., & Yao, Q. (2022). Estimation of subgraph densities in noisy networks. Journal of the American Statistical Association, 117, 361-374.
While it is common practice in applied network analysis to report various standard network summary statistics, these numbers are rarely accompanied by uncertainty quantification. Yet any error inherent in the measurements underlying the construction of the network, or in the network construction procedure itself, necessarily must propagate to any summary statistics reported. Here we study the problem of estimating the density of an arbitrary subgraph, given a noisy version of some underlying network as data. Under a simple model of network error, we show that consistent estimation of such densities is impossible when the rates of error are unknown and only a single network is observed. Accordingly, we develop method-of- moment estimators of network subgraph densities and error rates for the case where a minimal number of network replicates are available. These estimators are shown to be asymptotically normal as the number of vertices increases to infinity. We also provide confidence intervals for quantifying the uncertainty in these estimates based on the asymptotic normality. To construct the confidence intervals, a new and nonstandard bootstrap method is proposed to compute asymptotic variances, which is infeasible otherwise. We illustrate the proposed methods in the context of gene coexpression networks. Supplementary materials for this article are available online.