Meta-learning accelerates the learning process onunseen learning tasks by acquiring prior knowledgethrough previous related tasks. The PAC-Bayesiantheory provides a theoretical framework to analyzethe generalization of meta-learning to unseen tasks.However, previous works still encounter two notablelimitations:(l)they merely focus on the data-freepriors, which often result in inappropriate regular-ization and loose generalization bounds, (2)moreimportantly, their optimization process usually in.volves nested optimization problems, incurring significant computational costs. To address these issues, we derive new generalization bounds and introduce a novel PAC-Bayesian framework for meta-learning that integrates data-dependent priors. Thisframework enables the extraction of optimal posteriors for each task in closed form, thereby allow-ing us to minimize generalization bounds incorporated data-dependent priors with only a simple localentropy. The resulting algorithm, which employsSGLD for sampling from the optimal posteriors, isstable, efficient, and computationally lightweight,eliminating the need for nested optimization. Extensive experimental results demonstrate that ourproposed method outperforms the other baselines.
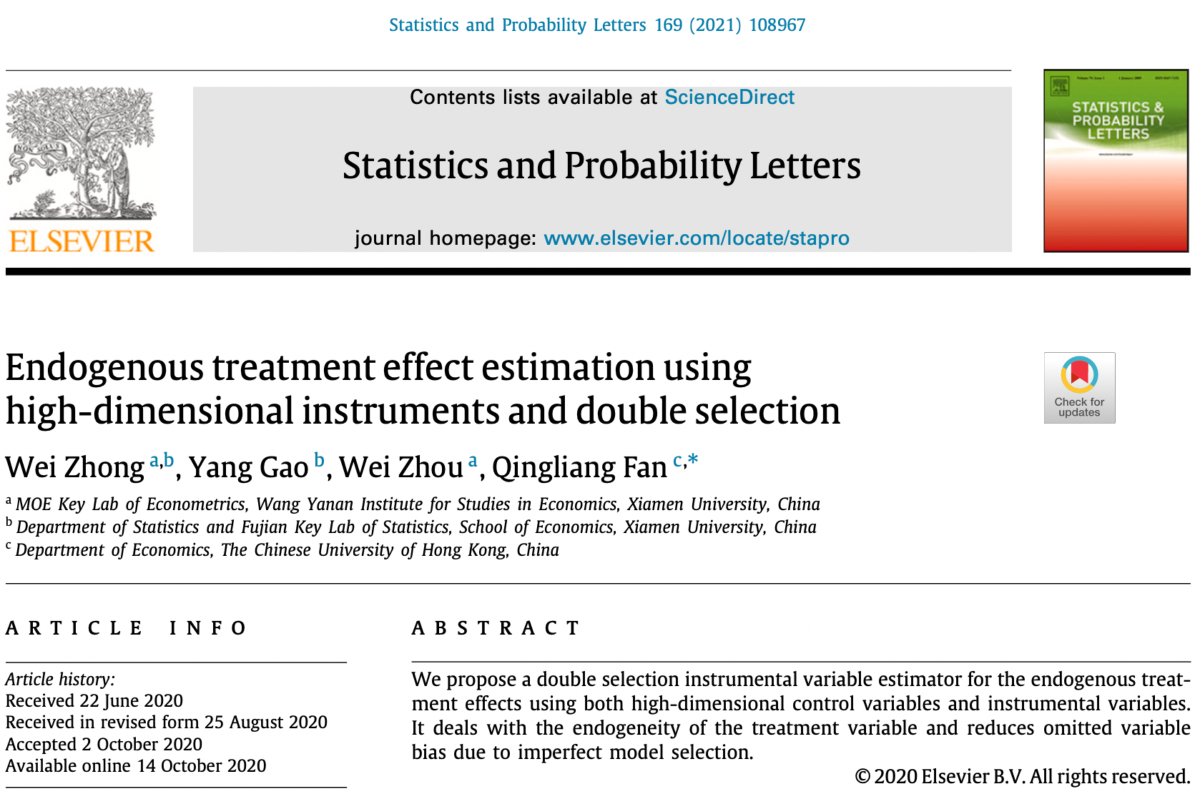