Sufficient dimension reduction is an important branch of dimension reduction, which includes variable selection and projection methods. Most of the sufficient dimension reduction methods are sensitive to outliers and heavy-tailed predictors, and require strict restrictions on the predictors and the response. In order to widen the applicability of sufficient dimension reduction, we propose BCov-SDR, a novel sufficient dimension reduction approach that is based on a recently developed dependence measure: ball covariance. Compared with other popular sufficient dimension reduction methods, our approach requires rather mild conditions on the predictors and the response, and is robust to outliers or heavy-tailed distributions. BCov-SDR does not require the specification of a forward regression model and allows for discrete or categorical predictors and multivariate response. The consistency of the BCov-SDR estimator of the central subspace is obtained without imposing any moment conditions on the predictors. Simulations and real data studies illustrate the applicability and versatility of our proposed method.
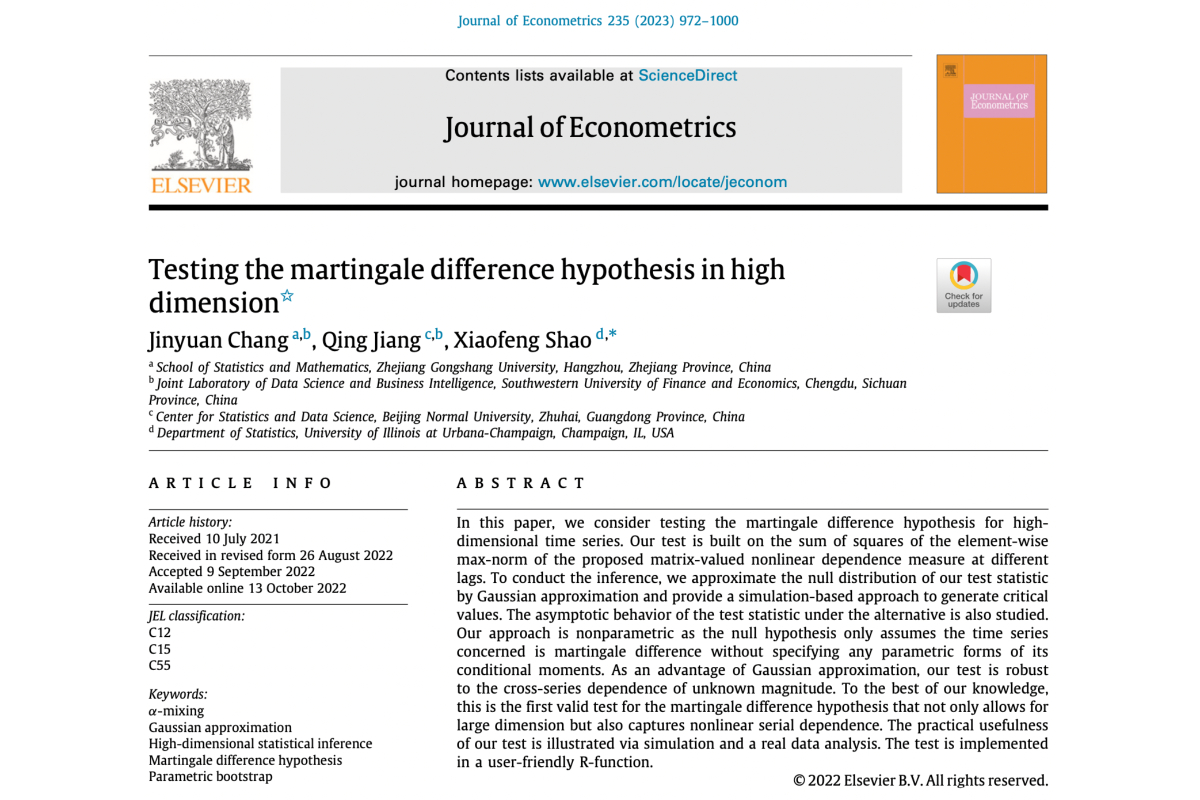